Dear B2C Growth Teams,
Feel free to bounce away, we’ve heard enough about your infinite backlog of experiments that flow into an infinite growing base of users to achieve significance in a matter of days.
Sincerely,
B2B Growth Teams
Okay, now that we got that out of the way we can chat about how statistical significance is so tricky in B2B SaaS. To start, your target market isn’t every consumer in the world; It’s a subset of businesses looking to solve a particular pain point. As a result, the users you have to experiment with decrease substantially as you move further down the funnel.
Previously, we used frequentist statistics when looking at sample sizes and evaluating our experiments.
Our flow:
Look at historical data (if we have it) to get a baseline conversion rate
Predict the % increase our experiment will have on that conversion rate
Plug this into Evan Miller’s sample size calculator
Build out the experiment and look at results once we hit that sample size
When starting a growth team at Jobber, we prioritized a scientific approach and made the conscious decision to hold ourselves accountable for proper experimentation. Statistical significance was at the core of decision making which left educated opinions on the sidelines.
Sounds good, right? One problem, our trusty sample size calculator continuously spits back a number of samples to reach 95% confidence that will take months to reach.
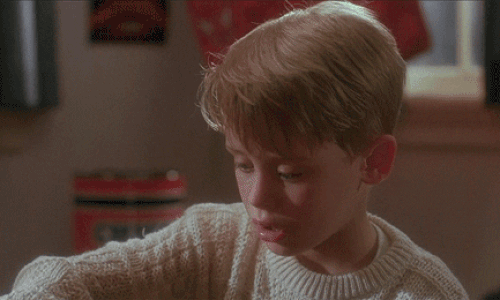
So, what does a savvy growth person do? Well, we increase the % lift we believe we will see in order for the sample size to get smaller, which in turn, gets us the thumbs up to go ahead and ship that experiment off into the wild.
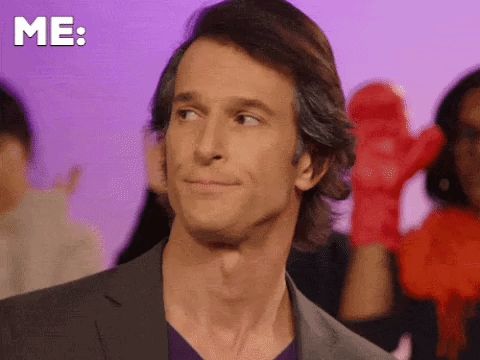
Raise your hand if you've ever played with a sample size calculator to fit a certain time frame?
Why is this bad? Well, you're now predicting a % increase that is unrealistic for this particular experiment. Therefore, your results will be non-significant and you will be sitting there unsure what to do next.
Generally, you try to find ways to build experiments that will see higher % increases to deal with areas of the funnel with low volume. Yet, we then miss out on opportunities for quick experimentation that lead to a collection of small wins that ladder up into an impactful output metric.
After a few long and drawn out experiments, we began looking at other approaches. Specifically, a shift into Bayesian statistics which welcomes an individual’s faith in the predicted result of the experiment. By adding this into the equation, we can make faster decisions in a shorter amount of time. This jumped us into a brand new shiny calculator.
Is it Perfect?
No! We’ve run into issues with positive results in our experiments with both Bayesian & Frequentist statistics. Software is always changing, markets adapt, and as a result, the positive results we see in experiments might not always hold true long term.
For example, one change sees a positive lift, but then a product team releases something new and marketing changes positioning. Suddenly, your change is now doing nothing for the business.
Experiments become a common suggestion but given all of these potential constraints, it’s important to reflect on whether or not you should be running an AB test. Tal Raviv has a great guide to help with this.
Our team continues to be data-focused, taking an experimental approach to growth opportunities. We leverage both frequentist & bayesian statistics pending what area of the funnel we are working in. Our main goal is continuous learning, and we accept the volume constraints we find ourselves in. Over time, it’s becoming less about what we can significantly prove and more about the risk we are willing to take on. In addition, we are working more on our qualitative research to better tool us with more information when making a decision in an area with volume constraints.